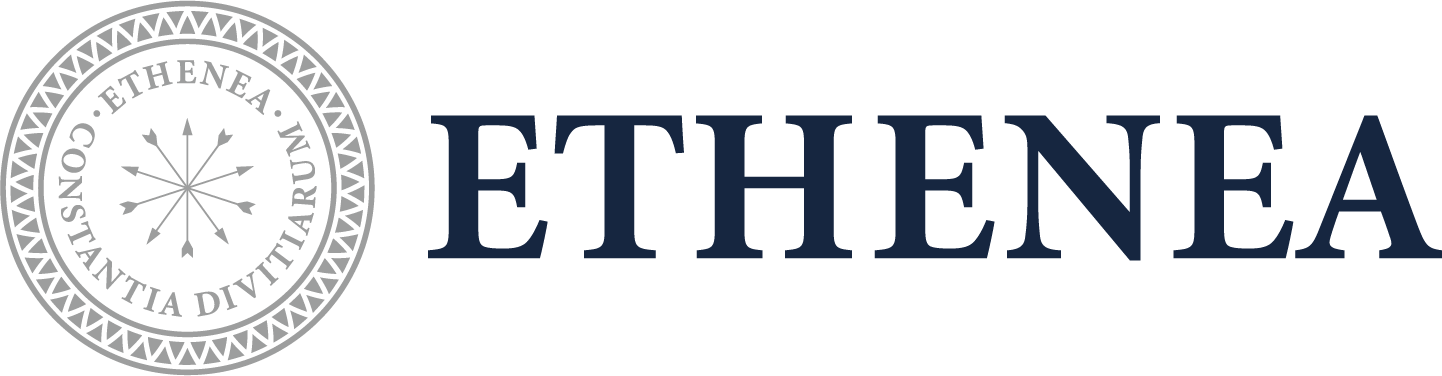
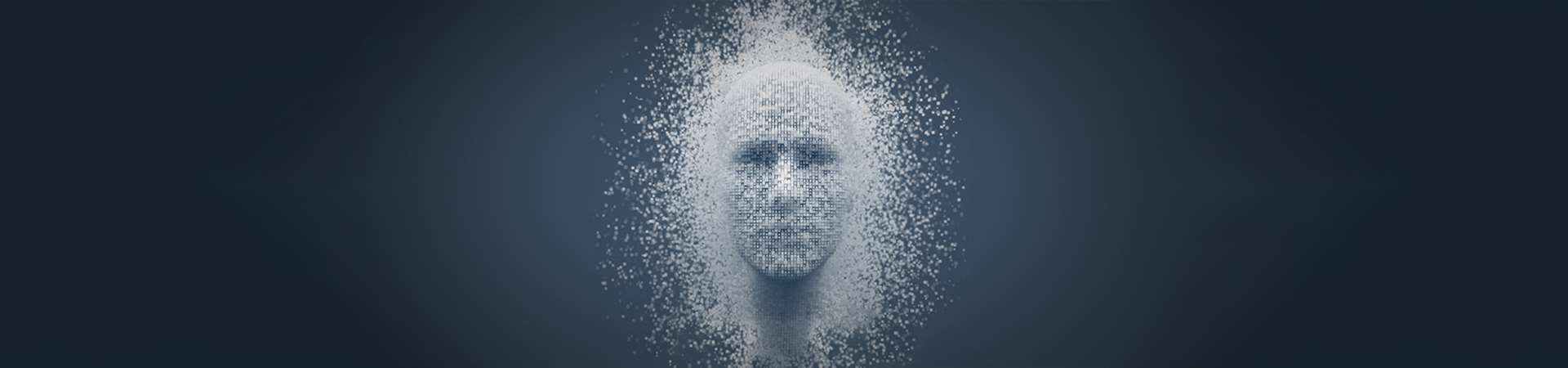
Why is alpha so elusive when we know of so many ways to generate it?
Key points at a glance
- The quest for excess return is probably as old as the capital markets themselves.
- Studies alone have identified hundreds of supposed sources. And the list of sources of alpha is getting longer all the time, as a study for ChatGPT showed in April.
- These means almost never produce the desired result. Instead, they harbour brand new risks for investors.
The article “ChatGPT Can Decode Fed Speak, Predict Stock Moves From Headlines”¹ was one of the most-read articles on financial news portal Bloomberg in April. Artificial intelligence in general and, specifically, the chatbot ChatGPT are also hot topics. The relentless quest for excess return, so-called alpha – returns in excess of the expected return based on market risk (beta) – is an ongoing theme that has been on the minds of almost all financial market participants for decades. So it’s no surprise that ChatGPT and other similar language models are now being used for these purposes. One of the first scientific papers on this subject, to which the first part of the heading of the aforementioned Bloomberg article refers, asks the exciting question in its title, “Can ChatGPT Forecast Stock Price Movements?”². The short answer is yes. At least, it can one day ahead.
The paper itself is worth reading just to get a sense of how to phrase the prompts you need to put into ChatGTP; along the lines of “[…] Pretend you are a financial expert. You are a financial expert with experience in stock recommendations. Tell me […]” – or to get interesting insights into the highly standardised data on price movements and, in particular, news reports that make such studies possible in the first place. However, this commentary is not so much about the specific results of backtesting from the paper (there will no doubt be much more spectacular results to be examined in the course of the next few months and years) than it is about determining more generally what direct benefit you as an investor or we as an investment company can glean from the latest findings.
Our guess is more or less the same benefit that you, we and all other investors got from the more than 400(!) scientifically proven factors for excess returns documented in financial magazines up to 2019. The two financial researchers Campbell R. Harvey and Yan Liu compiled a comprehensive list³ with an overview of all publications. Interested readers can look up the individual factors.
“The rate of factor production
in the academic research is out of control.”
Campbell R. Harvey and Yan Liu in “A Census of the Factor Zoo” (2019)⁴
Admittedly, after a few years of experience in capital markets, it is not easy to keep a straight face when speaking of the plethora of possible sources of excess return. Alpha has always been a very delicate flower and also very short-lived. And that will always be the case. The discovery of supposed new sources of alpha – which has increased exponentially of late due for one to the improvement in the technological possibilities – will do nothing to change that. There have also been studies that show that the effect of such alpha characteristics lessens substantially once they are published. The paper “Does Academic Research Destroy Stock Return Predictability?”⁵ published in 2015 provides a good overview of this. It also describes that, once found, an outperformance will diminish all the faster the more attractive it was at the time of discovery and the easier it is to put that strategy into practice. In short, the search for ever new sources of alpha heats up a race that is almost impossible to win with these very means.
Sometimes we are also personally automatically assumed to operate in this corner of the market. This happens to me relatively often as a financial mathematician, in which capacity I write this commentary. This is why, when introducing myself, after I state my qualification, I routinely add that “while I’m good with numbers, I find more risks than opportunities in quantitative-based investment strategies.” The constant weighing up of potential opportunities against potential risks is ultimately the kernel of our job as active asset managers. When I am talking to people, I like to give an example from the early years of my career. It illustrates the underlying issue very well and has been repeated countless times in a similar vein in the market since then and there is every likelihood that it will be repeated in the coming years with investment products labelled “artificial intelligence”.
The actual name and provider of this market-neutral product are not important here. The investment approach used and the underlying technique were state-of-the-art not quite 20 years ago and, with a size of almost EUR 10 billion at its peak, it wasn’t a niche product either. The investment strategy can be summed up, in highly simplified terms, as “buy the prospectively most attractive widely diversified equities in the market and sell the prospectively most unattractive equities in the market”. The market risk (beta) was effectively zero, so one could concentrate fully on continuously siphoning off alpha. The strategy worked phenomenally well at the start and delivered constantly positive performance without major fluctuations in price. Just like the theory and product blurb predicted:
Figure 1: Performance in the first eight months after product launch
Source: Bloomberg L.P.
What happened a short time later fell far short of all expectations:
Figure 2: Performance in the first ten months after product launch
Source: Bloomberg L.P.
While on the surface of the market everything was relatively calm, the fund suddenly lost value day after day to the tune of the aggregate performance of multiple months. Investors were horrified and perplexed. What had happened? Using the new technological means and data many market participants had analysed the markets to find out which equities produced the easiest and most reliable excess returns in hindsight. Unsurprisingly, because the same data were examined with the same means, the same results were arrived at. Many investors simply had the same securities in their portfolios. As soon as investors had to sell to any appreciable extent, the shares came under pressure and a chain reaction was triggered. It was an important and instructive experience for many investors, which went down in the capital market history books under the chapter heading Quant Crash 2007.
“Unsurprisingly, Because the same data
were examined with the same means,
the same results were arrived at.
And thus the same performance.”
Christian Schmitt
For the sake of full disclosure, here’s what happened next in our example. Some of the losses were very quickly recouped in the first countermovement. The strategy was tweaked based on the lessons learned and made it through the crisis year of 2008 very well. Thereafter, however, it went into steady decline, without ever being able to get close to matching the successes of the backtesting or of the first few months and fulfilling the ambitions it proclaimed. After around eight and a half years, in 2015, the product that was once worth billions was taken off the market.
Figure 3: Complete life cycle of the product
Source: Bloomberg L.P.
The example given above is instructive and helpful in many respects. It shows clearly that the herd mentality of investors presents a risk that cannot be underestimated not only at overall market level but also at individual security level. Interestingly, this risk does not correlate with traditional risk factors such as debt, valuation, cyclicality, beta and others. For that reason, it is infinitely more difficult to protect oneself from these positions referred to as crowded trades. While diversification of the portfolio essentially protects the portfolio, it is the investment strategy itself that harbours the potential risk here. Particular caution is thus advised in the case of both very popular investment strategies and new, innovative approaches that promise above-average returns and – therefore attract lots of investment – on the back of fresh backtesting, newly discovered alpha factors or new technological possibilities.
In addition to these immediate risks that arise at very short notice, in the medium to long term this example shows how quickly sources of alpha dry up. Practice and theory show that this tends to be the rule rather than the exception.
What does this mean in concrete terms for us in ETHENEA? Sure, headlines like “ChatGPT can predict share price movements” are intriguing but, for the reasons outlined, they don’t send us into either a panic or raptures. That said, new tools such as ChatGPT will permanently change the work of all market participants and investors. Just like pocket calculators, computers and the internet before it. To assess opportunities and risks going forward it is therefore essential to understand the evolution and what the potential implications of this development will be. This first financial paper on ChatGPT shows that at least one existing trend in the financial industry is being reinforced: the focus on ever shorter trading intervals, where day-to-day investments based on headlines no longer provoke any head-shaking to speak of. Perhaps the value of active management today consists in reading the story behind the headline. Let’s see if this is declared an official source of alpha!
¹ https://www.bloomberg.com/news/articles/2023-04-17/chatgpt-can-decode-fed-speak-predict-stock-moves-from-headlines
² https://ssrn.com/abstract=4412788
³ https://docs.google.com/spreadsheets/d/1mws1bU56ZAc8aK7Dvz696LknM0Vp4Rojc3n61q2-keY/edit?usp=sharing
⁴ https://ssrn.com/abstract=3341728
⁵ https://ssrn.com/abstract=2156623
Please contact us at any time if you have questions or suggestions.
ETHENEA Independent Investors S.A.
16, rue Gabriel Lippmann · 5365 Munsbach
Phone +352 276 921-0 · Fax +352 276 921-1099
info@ethenea.com · ethenea.com